Abstract
Data integration brings data together from different systems to increase its value to any business. Without data integration, there is no way to access the data gathered in one system from another, or to combine data sets so they can be processed, analysed and acted on. Data also is the foundational enabler of end-to-end automation, a hallmark of all digital companies and a huge boost to customer experience, faster time to market, working within an ecosystem and operational efficiency, among other things.
Importantly, data integration helps to clean and validate the data that businesses rely on. Companies need their data to be robust, free of errors, duplication, and inconsistencies, which is a big ask when a company is running maybe hundreds of databases with the data often held in silos and incompatible formats.
It becomes even more of a challenge as the extended enterprise becomes increasingly common – that is companies using a platform-based ecosystem to provide products and solutions with partners in B2B and B2B2X business models. In this operational model, companies also need to offer their data to third parties in a secure, controlled and timely way.
Companies need a proper integration strategy to make their data more usable and relevant, avoiding pitfalls such as heavily customized integrations that fit today’s needs but ossify rapidly and become obstacles when flexibility and fluidity in data’s use are what’s required.
This white paper looks at what data integration is and the various tools and approaches that are available for future-proof data integration – such as APIs, data virtualization, containerization and microservices, cloudification, analytics and business intelligence, and working with a data integration specialist firm such as Torry Harris Integration Solutions (THIS). It concludes with critical success factors.
Introduction
One of the defining characteristics of a digital company is that it bases decisions on information and intelligence derived from data. Yet this apparently straightforward principle of using data to inform business and operational decisions is anything but simple to follow. This is because of the volume, variety, velocity and veracity aspects shown below. Also, the greatest value is derived from combining data sets to extract value and insights – hence data integration is a multi-faceted and complex discipline.
Volume
Companies generate immense amounts of data from sources including their networks, services and customers, as well as gather it from other sources like social media |
Variety
The data comes in many formats, is often incomplete, siloed, incompatible, structured and unstructured. |
Veracity
Data should be a ‘single source of truth’ – the cleansing needed to get it to that point is not necessarily simple. |
Velocity
We need the speed that data is produced at rises all the time. The question is but how fast a firm can act on it in real time or close to it. |
Value is derived from integrating data to gain insights, improve CX, efficiency, profitability & TTM
|
It is becoming more complex as the extended enterprise becomes increasingly common – that is companies using a platform-based ecosystem to provide products and solutions with partners in B2B and B2B2X business models. They need to expose their own data and consume data from others to interoperate successfully.
It’s worth noting that when data integrations fail, it is rarely solely due to technical issues so much as poor planning, strategy and execution – and sometimes tools can seem more of a hindrance than a help. The starting point always has to be for enterprises to have a clear vision of what they want to achieve through data integration, and resist the temptation of a shiny new piece of technology providing the reason to kick off a project.
After the vision, comes the strategy to make it happen, and other preparatory steps. For instance, the quality of data is critical – garbage in, garbage out applies here just like everywhere else. Companies need to think about data cleaning – removing records that are incomplete, duplicated, out of date and so on – before they start integration.
The data lifecycle
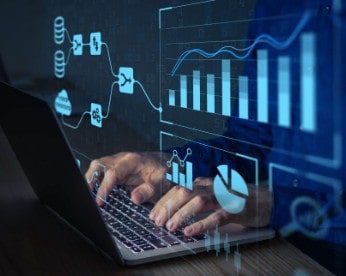
It is also crucial to see data integration as part of the wider transformation canvas. It cannot be viewed in isolation. That custom integration of two monolith systems might work really well now, but what happens when business needs change and the data must be exposed and combined with other systems’ or parties’ data sets? That customization could become a most inflexible barrier?
Never lose sight of the fact that data is the key enabler of automation – another hallmark of a digital company – and that goal is end-to-end automation, not isolated automated islands, to deliver operational efficiency, the best customer experience, shorter time to market, and increased profitability.